In the realm of scientific research, p-values play a crucial role in assessing the significance of experimental results. These probabilities, often mentioned by scientists, hold valuable insights into the validity and reliability of their findings. In this comprehensive guide, we will delve into the world of p-values, shedding light on their definition, interpretation, and the impact of random noise. By the end, you’ll have a clear understanding of what p-values truly signify and their role in scientific investigations.
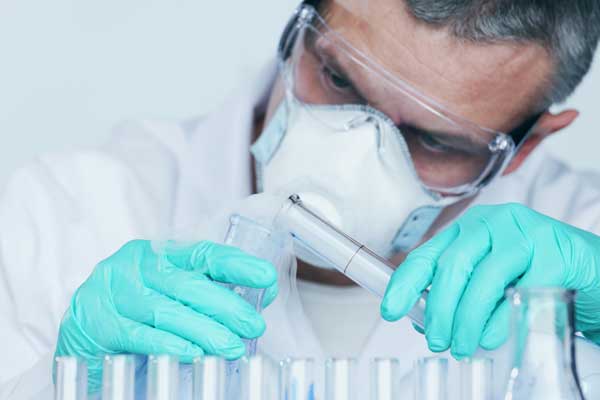
What is a P-Value and How Does It Work?
The first step in unraveling the mystery behind p-values is understanding their fundamental definition and purpose. We’ll explore the concept of a p-value as a probability value, with a range between 0 and 1. Through an example, we’ll illustrate how this numeric representation relates to the observed difference in an outcome measure when no difference exists between treatments in the population. This section will clarify the essence of p-values and their significance in scientific experiments.
The Null Hypothesis and Its Role in P-Values
To comprehend the full extent of p-values, we must acquaint ourselves with the concept of the null hypothesis. We’ll explain its definition and significance, particularly in the context of comparing treatment groups. By examining a weight-loss drug experiment, where one group receives a placebo and the other the experimental drug, we’ll showcase how the null hypothesis assumes no difference between the two groups. Understanding the null hypothesis lays the foundation for grasping the role of p-values as evidence against it.
Interpreting P-Values: Strength of Evidence
Now that we’ve established the groundwork, it’s time to decipher the meaning behind p-values and their interpretation. We’ll delve into the analogy of a court trial, where the null hypothesis serves as the defendant. By grasping the relationship between p-values and the probability of observing a difference as large or larger than the one in the sample, we’ll gain insights into the strength of evidence against the null hypothesis. This section will demystify the significance of p-values in scientific decision-making.
Statistical Hypothesis Tests: Unveiling the Process
To obtain p-values, scientists employ statistical hypothesis tests. In this section, we’ll provide an overview of common tests such as the student t-test and one-way ANOVA. While avoiding overwhelming statistical jargon, we’ll shed light on the process involved in obtaining a p-value from collected data. By understanding the mechanics behind statistical hypothesis testing, readers will develop a clearer picture of how p-values are derived and their role in confirming or challenging scientific hypotheses.
Random Noise: Unraveling the Impact on P-Values
One crucial factor that affects the interpretation of p-values is random noise, which encompasses various elements that introduce uncertainty into experimental results. We’ll explore the concept of random sampling and its potential impact on p-values, particularly in human studies. Using the weight-loss drug experiment as an example, we’ll delve into the coincidences of random sampling and how they can skew the results. This section highlights the importance of acknowledging and accounting for random noise when interpreting p-values.
Conclusion
In conclusion, p-values serve as a powerful tool in the realm of scientific research, providing valuable insights into the significance of experimental outcomes. Through an exploration of their definition, interpretation, and the impact of random noise, we have gained a comprehensive understanding of the role p-values play in scientific investigations. Armed with this knowledge, researchers and enthusiasts alike can navigate the intricate world of statistical hypothesis testing with greater clarity and confidence.